Premium
Data Science with R Training Course
The Data Science with R programming certification training covers data exploration, data visualization, predictive analytics, and descriptive analytics techniques with the R language. You will learn a...
- All levels
- English
Course Description
The Data Science with R programming certification training covers data exploration, data visualization, predictive analytics, and descriptive analytics techniques with the R language. You will learn about R packages, how to import and export data in R, data structures in R, various statistical concepts, cluster analysis, and forecasting. The training on Data Science with R provides the skills requ...
The Data Science with R programming certification training covers data exploration, data visualization, predictive analytics, and descriptive analytics techniques with the R language. You will learn about R packages, how to import and export data in R, data structures in R, various statistical concepts, cluster analysis, and forecasting. The training on Data Science with R provides the skills required to work with real data sets and provide an opportunity to use data to provide data-driven strategic and tactical recommendations. This training will provide some insights on techniques such as linear and logistic regression, ANOVA, Segmentation, Ensemble models, SVM and machine learning in big data. In addition to technical skills, the program also allows students to build effective leadership and communication skills to advance their career upon graduation. The Data Science with R covers data exploration, data visualization, predictive analytics, and descriptive analytics techniques.
What you’ll learn
- Live Class Practical Oriented Training
- Timely Doubt Resolution
- Dedicated Student Success Mentor
- Certification & Job Assistance
- Free Access to Workshop & Webinar
- No Cost EMI Option
- Explore R data structures and syntaxes
- Read and write data from a local file to a cloud-hosted database
- Work with data, get summaries, and transform them to fit your needs
- Work with data, get summaries, and transform them to fit your needs
- Explore R language fundamentals, including basic syntax, variables, and types
- Explore R language fundamentals, including basic syntax, variables, and types
- Create functions and use control flow
- Learn to program in R at a good level
- Learn how to build and use matrices in R
- Understand the Normal distribution
- Foundational R programming concepts such as data types, vectors arithmetic, and indexing
- How to perform operations in R including sorting, data wrangling using dplyr, and making plots
Curriculum
Frequently Asked Questions
This course includes
- Duration 160 Hour
- Language Hinglish
- Certificate Yes
Education Provider
More Courses
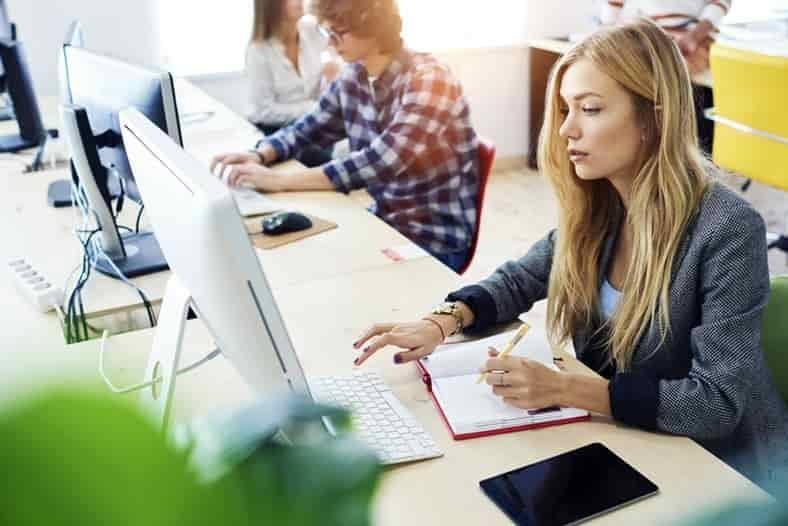
3D Printing Training Cour.
- ₹ 5000
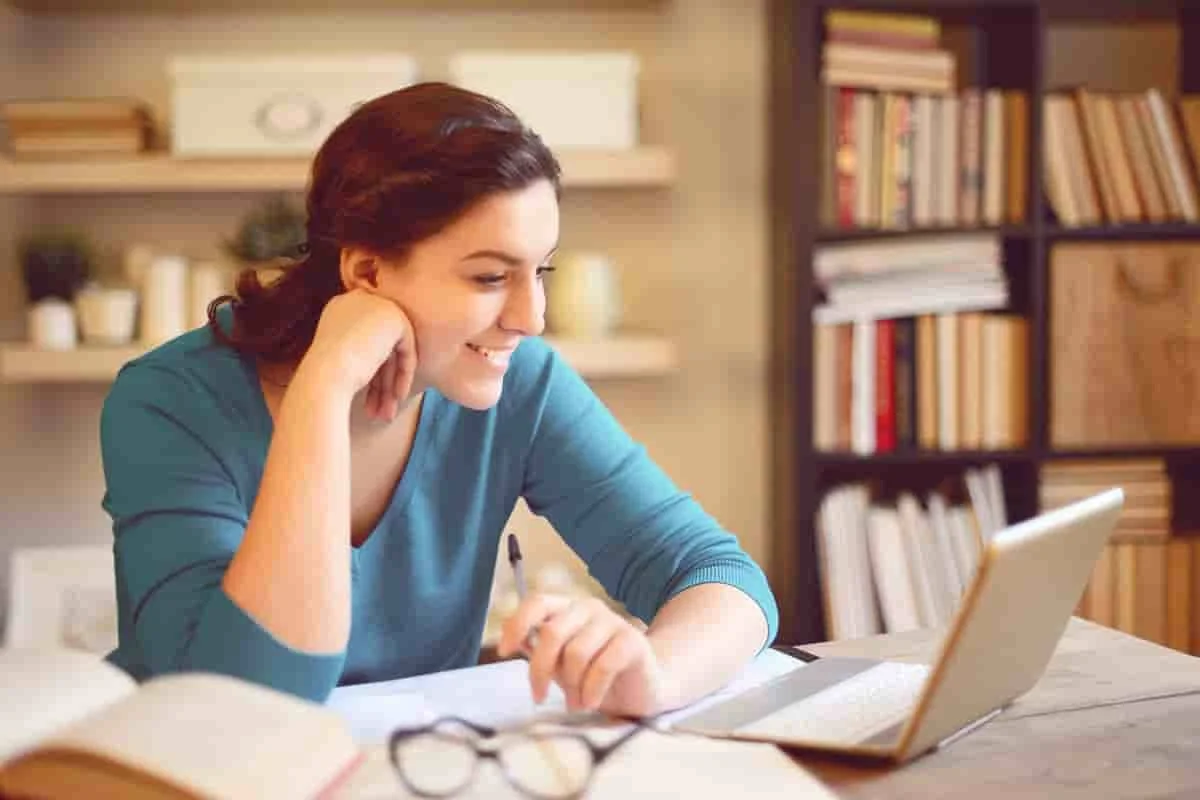
3ds Max Training Course
- ₹ 20000
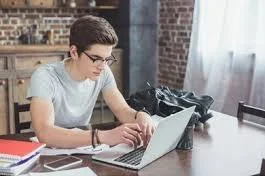
AI & Deep Learning with T.
- ₹ 40001
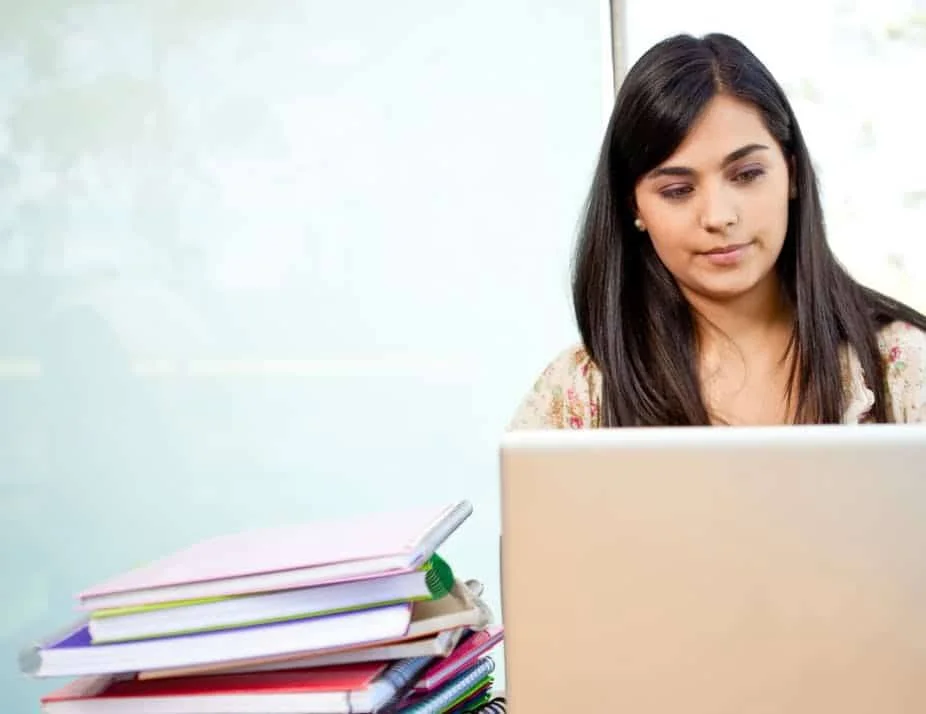
ASP.NET Core Live Project.
- ₹ 24999
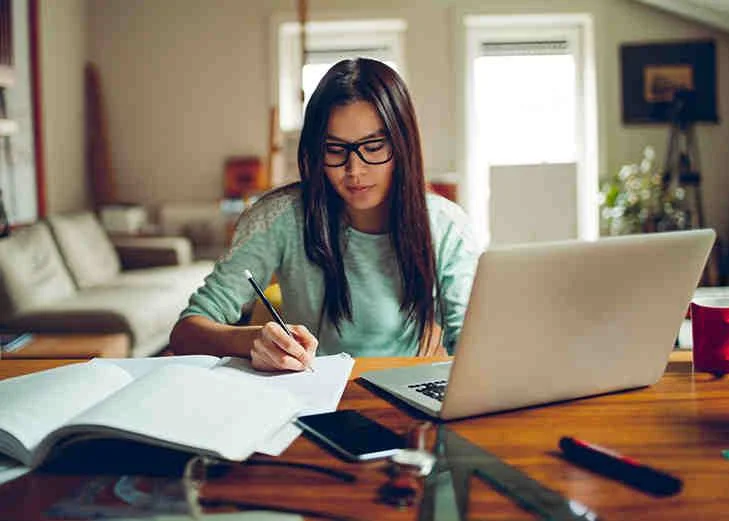
ASP.NET Live Project Trai.
- ₹ 45000